This article is an overview of the current state of the game based on statistical analysis of Star Wars Legion game data.
In my professional life I am a research scientist and have always thought about applying rigorous statistical analysis to Legion data. When hearing the opinions of talking heads and general community sentiment, I have wondered what is actually supported by the numbers? The one issue was the data was never available or curated, so doing something like this was always just a pipe dream. Then came along Legion Stats which is a repository for legion tournament data. Because we now have this resource of raw Legion data with thousands of recorded games, I finally had enough numbers to satisfy my desires as a data scientist. This article is meant to be an overview of the current state of the game based on statistical analysis, hence the name “State of the Legion”. Because we now have Legion data readily available, I plan to do a “State of the Legion” article regularly. This first article will focus on quantifying the effects of Battle Forces and Shadow Collection on Legion. If there are questions you are interested in, please leave a comment. I plan to add different analyses to future iterations. I also want to thank the community members and tournament organizers who have been working hard to collect lists from their players and submit them in a format we can import to Legion Stats. It was amazing having events like the Hannover World qualifier run by Finn P which had over 70 players. This event had every list submitted in a format LegionStats could automatically import. You can find the guidelines for list submissions here, but essentially just make the first line of your list submission a link to Legion HQ or Tabletop Admiral. List data submitted as an image or as text cannot be imported automatically. This really helps us import the data effortlessly, otherwise it takes hours to days of manual curation to upload the data (I am looking at you Gencon). Now onto the fun stuff!
You may be asking “why statistical analysis?” The main reason for doing something like this is to try and remove personal bias and see what the numbers tell you. While fully removing the bias of the author is impossible, basing your interpretation on a statistical quantification helps reduce this bias. Despite this effort the discussion and conclusions presented here are still a synthesis of the authors interpretation of the statistical results, study design, and personal anecdotal evidence. While statistics can provide support for an interpretation or conclusion you can never truly “prove” anything with a statistical analysis. Because of this, as with all research, I would encourage the reader to draw their own conclusions.
Now I know most people are not familiar with the format of research papers, so I have a brief outline below of what to expect. Not everyone is interested in all the details, so this article is split up into different sections so you can skip around depending on your interest level. If you want the big takeaways just read the abstract and discussion, if you want all the nitty gritty details you can read the entire article, and if you want to know exactly how everything was done you can also read the linked supplemental documents.
Outline
- Abstract (Too long didn’t read, this is a short summary of everything here)
- Introduction (Background and the motivation for the analysis)
- Results (The data and statistical results, just the hard facts)
- Discussion (Putting the results in context, and my interpretation)
- Conclusion (Closing summary and implications for the future)
- Supplements (Linked document with explicit methods explaining exactly how the data was collected, how the analysis was performed, and the raw data itself. Anything citied with an S in front of it i.e.: STable 1 is found in the supplements)
Abstract
This analysis was done in order to quantify the effects of the release of the Shadow Collective and Battle Forces on Star Wars Legion. This release was one of the largest in the history of the game, creating an exciting new increase of content. Legion has also grown in popularity over the last year resulting an abundance of game data. This data created the opportunity for a rigorous statistical analysis of the state of the game. The State of Legion was quantified based on tournament data with a focus on inter-faction balance and the effects of army unit composition. The analysis revealed the release of Battle Forces created a previously absent imbalance between factions. This statistically significant imbalance was primary driven by the Shadow Collective displaying an unprecedented level of dominance while the Republic significantly underperformed compared to other factions. Analysis of unit composition revealed all units within the Shadow Collective have a significant positive effect on win rate while all Republic exclusive units had a significant negative effect on win rate. The Empire, Rebels, and Separatists showed mixed results, but displayed relative balance between them. I compared the dominance of the Shadow Collective to previously over tuned archetypes (Clone Standby Sharing, and Clone Gunlines aka Rexstar) and found the Shadow Collective outperformed these archetypes across the board. This analysis suggests that Shadow Collective and Republic may need to be the focus of future balance updates in order to bring back competitive balance.
Introduction
We had two massive releases for the game of Legion recently. First Mercenary units and the Shadow Collective battle force were introduced to the game. This Battle Force functions similarly to a faction where you can run all mercenary units (besides Boba Fett). This release was the largest wave since the Clone Wars factions were added. Shortly after, Battle Forces were introduced for the already existing factions: Blizzard Force (Empire), Echo Base Defenders (Rebels), 501st Legion (Republic), and the Separatist Invasion Force (Separatists). In the span of one week, the game received two massive updates to the game. Needless to say, the state of the game shifted overnight, creating an exciting opportunity to explore the new meta.
Because of this massive change I sought to quantify the impact of these new releases and figure out what the new meta looks like. I first asked what was the effect of Battle Forces and Mercenaries on inter-faction balance. While the ideal scenario would be perfect balance between all factions and internal balance within factions, I believe a more realistic goal is at least balance between factions. This would mean regardless of what faction you collect you will always have at least one competitive option to play. Second, I asked what was the effect of unit composition on win/loss rates. This was an effort to try and identify which units are the most competitive in the current meta and which units are good candidates for changes in future points adjustments or reworks. With this analysis we can help determine which archetypes in each faction will be most successful and get an idea about faction internal balance.
Results
To quantify the effect the introduction Battle Forces and Mercenaries had on faction balance I compared win and loss counts between factions both before (1/Jan/2022 – 30/Jun/2022, Post 2021 points update and Kashyyyk wave) and after the release of Battle Forces (1/Jul/2022 – 22/Aug/2022). I then compared win and loss counts based on presence of various units and activation number. In this article, all statistics were performed on events after the release of Battle Forces and Mercenary units (1/Jul/2022 – 22/Aug/2022) unless otherwise explicitly stated.
Differences in win rates in the pre and post Battle Force metas revealed a shift in faction balance. There was a significant difference in faction win and loss counts after the release of Battle Forces and Mercenaries (Pearson’s Chi Square p-value = 0.008)(Fig 1A, Stable 1). This is in contrast to no significant effect of faction on win and loss counts before the release (Pearson’s Chi Square p-value = 0.114)(Fig 1B, Stable 2). In particular the Mercenary faction (Battle forces with no parent faction are categorized as Mercenary, currently only Shadow Collective) out preformed all other factions with a 63% win rate, and Republic underperformed all other factions with a 43% win rate. After Mercenaries, Empire had the second highest win rate at 52%, followed by Rebels at 49% and Separatists at 46% (Fig 1A, STable 1). The biggest change in win rate between pre and post battle force release was Separatists (Win rate Δ = -8%) dropping from 54% to 46%, followed by the Republic (Win rate Δ = -4%) dropping from 47% to 43% (Fig 1, STable 1, STable 2). Overall, the introduction of Battle Forces resulted in a statistically significant imbalance in faction win rates that was not present before the release of Battle Forces.
I next quantified the effects of unit composition on win and loss counts. I tested the effect of activation number and units present in an army separately. Although there was no significant effect of activation count on win rates (Pearson’s Chi Square p-value = 0.120) 8-9 activations had a below average win rate (43-46%) while 10-11 activations had an above average win rate (53%)(Fig 2, STable 3). 12 Activations had a high win rate of 66% but had a small sample size of only 27 total games.
When comparing the effect of units present in an army, we found unit composition had a significant effect on win rates (Pearson’s Chi Square p-value = 7.9*10-5). Units were ranked based on their residual effect on win counts to show which units had the largest positive and negative effect on win rates (Table 1, Table 2, STable 4). The residual effect considers both the sample size and magnitude of the positive or negative win rate. For example, a unit with a high win rate but few games played may be ranked lower than a unit with a lower win rate but more games played. Patterns of units with less games have a statistically higher chance of being a result of random effects, so this analysis takes that likelihood into account. I collected the top 15 units (Table 1) and bottom 15 (Table 2) units to capture the main units that are both over performing and underperforming currently. You can find the full list of units included and their residual rank in the supplements along with a list of units excluded from the analysis since they had less than 20 games played (STable 4, 5).
Rank | Unit | Wins | Losses | Win rate | Total games | Residual |
---|---|---|---|---|---|---|
1 | Imperial Officer Ruthless Commander | 83 | 45 | 64.84% | 128 | 2.275 |
2 | Maul A Rival | 60 | 30 | 66.67% | 90 | 2.151 |
3 | Pyke Syndicate Foot Soldiers | 110 | 71 | 60.77% | 181 | 1.935 |
4 | A-A5 Speeder Truck | 45 | 23 | 66.18% | 68 | 1.813 |
5 | Pyke Syndicate Capo | 98 | 64 | 60.49% | 162 | 1.781 |
6 | Black Sun Enforcers | 58 | 34 | 63.04% | 92 | 1.686 |
7 | Stormtroopers Heavy Response Unit | 23 | 10 | 69.70% | 33 | 1.547 |
8 | Snowtroopers | 52 | 33 | 61.18% | 85 | 1.378 |
9 | Cad Bane Needs No Introduction | 63 | 42 | 60% | 105 | 1.362 |
10 | Darth Vader The Emperor’s Apprentice | 48 | 34 | 58.54% | 82 | 1.018 |
11 | Bossk Trandoshan Terror | 60 | 46 | 56.60% | 106 | 0.877 |
12 | T-Series Tactical Droid Programmed For Strategy | 32 | 23 | 58.18% | 55 | 0.796 |
13 | Boba Fett Infamous Bounty Hunter | 39 | 29 | 57.35% | 68 | 0.789 |
14 | 74-Z Speeder Bikes | 42 | 32 | 56.76% | 74 | 0.751 |
15 | Darth Vader Dark Lord of the Sith | 18 | 12 | 60% | 30 | 0.728 |
Out of the top 15 units with the biggest positive effect on win rates, 9 out of the 15 (9/15) units either released or were updated in the Battle Forces and/or Shadow Collective waves. Of these top 15, 12/15 could be played in Empire, 7/15 in Shadow Collective, 6/15 in Separatists, 3/15 in Rebels, and 2/15 in Republic (Table 1). The only units playable in Rebels and Republic in the top 15 were mercenary units (Pykes, and the A-A5). The only non-mercenary unit for Separatists was the T-Series Tactical Droid. Empire had the most non-mercenary units in the top 15 and the Imperial Officer had the largest positive residual effect on win rate out of any unit in the game.
Rank | Unit | Wins | Losses | Win rate | Total games | Residual |
---|---|---|---|---|---|---|
1 | Maul Impatient Apprentice | 17 | 31 | 35.42% | 48 | -1.474 |
2 | Imperial Special Forces | 17 | 30 | 36.17% | 47 | -1.386 |
3 | Super Tactical Droid Kalani | 29 | 44 | 39.73% | 73 | -1.3 |
4 | DSD1 Dwarf Spider Droid | 25 | 39 | 39.06% | 64 | -1.292 |
5 | Phase I Clone Troopers | 74 | 96 | 43.53% | 170 | -1.287 |
6 | T-47 Airspeeder | 15 | 26 | 36.59% | 41 | -1.257 |
7 | Phase II Clone Troopers | 29 | 43 | 40.28% | 72 | -1.226 |
8 | Padme Amidala Spirited Senator | 41 | 57 | 41.84% | 98 | -1.213 |
9 | Wookiee Warriors | 37 | 52 | 41.57% | 89 | -1.191 |
10 | AT-RT | 18 | 29 | 38.30% | 47 | -1.181 |
11 | DRK-1 Sith Probe Droids | 10 | 18 | 35.71% | 28 | -1.104 |
12 | ARC Troopers Strike Team | 32 | 43 | 42.67% | 75 | -0.96 |
13 | Imperial Death Troopers | 27 | 37 | 42.19% | 64 | -0.941 |
14 | Sabine Wren Explosive Artist | 12 | 19 | 38.71% | 31 | -0.927 |
15 | B2 Super Battle Droids | 22 | 31 | 41.51% | 53 | -0.925 |
There were no mercenary units in the 15 units with the largest negative effect on win rate (Table 2). Of the bottom 15, 5/15 were Separatist, 4/15 were Rebel, 4/15 were Republic, and 2/15 were Empire. Notably the previously dominant Maul Impatient Apprentice, Imperial Special Forces, Super Tactical Droid Kalani, DSD1 Dwarf Spider Droids, and DRK-1 Sith Probe Droids all landed in the bottom representing a stark shift in the meta. Republic was the only faction without a single unit with a positive win rate (Excluding Pykes)(STable 4). Out of the 22 units excluded from this analysis for having less that 20 games played, 7 were Rebel, 5 were Separatist, 5 were Empire, and 5 were Republic (STable 5).
Discussion
Current Faction Differences
One of the first things that jumped out of the analysis was the faction Imbalance that came with the release of Battle Forces. While there were differences in faction-by-faction win rates after the 2021 RRG update, these differences were not statistically significant (Figure 1B). After Shadow Collective and Battle Forces were introduced, the data showed a much larger and statistically significant discrepancy between faction win rates (Figure 1A). Currently Shadow Collective is outperforming every faction with a massive 63% win rate (STable 1). The top performing faction before Battle Forces was the Separatists with a 53% win rate (STable 2). To put this win rate into context, we have never observed a faction win rate this high since the inception of the game. The two closest instances were the Republic during standby sharing at 58% (Stable 6, Invader League 5), and the Republic post standby nerf but pre 2021 points adjustment with 56% (STable 7, Invader League 6). It is worth noting all units in the Shadow Collective roster have positive win rates and 7 out of the 10 units currently in the faction are in the top 15 units that have a positive effect on win rate (Table 1). Essentially everything in the Shadow Collective is hyper competitive; there are no “bad” Shadow Collective archetypes currently.
At the other end of the spectrum, Republic win rates have continued to decrease after the release of Battle Forces. The Republic had a win rate of 47% before Battle Forces, which was not significantly different from the other factions (Figure 1B). After Battle Forces and the Shadow collective were released the win rate of the Republic dropped to 43% which was a statistically significant difference in win rate compared to the other factions (Figure 1A). This is the second lowest win rate in the history of the game. The only other faction with a lower win rate was Empire during the Clone Gunline meta (Rexstar) post standby nerf with a 35% win rate (STable 7, Invader League 6). The Republic has the exact opposite issue as the Shadow Collective; every unit in the Republic has a negative effect on win rate and the only units with a positive effect are Pykes (Table 2, STable 4). In addition, it seems Battle Forces are not helping the Republic either. We did not have a single recorded game of the 501st Legion. This reflects community sentiment that the 501st Legion is not competitively viable, but also shows a general disinterest in this Battle Force in its entirety.
The other three factions, Empire, Rebels, and Separatists had slight differences in win rates that were not statistically significant. Within these factions we saw variation in the success of units with certain archetypes outperforming others (STable 4). This data together suggests these factions are currently balanced against each other and the Shadow Collective and Republic are the two factions causing the imbalance between factions. In the next section I will go into more detail on each faction individually and the path forward competitively.
The Four Horsemen of the Apocalypse
I know the name of this section is hyperbolic but there are 4 lists that stand out, so I couldn’t resist.
Shadow Collective Pyke Spam
This army leans into the power of Pykes and is a deadly efficiency gunline. There are different flavors of this army some including a bounty hunter like Bossk, or Black Sun Enforcers. This version in particular was played by Matt Bronson at Lone Star Open 2022 where he finished in 1st. The army also brings Maul as a play maker focus piece who can score objectives while the rest of the army shoots everything off the board. Every unit in this army is in the top 15 units that have a positive effect on win rate (Table 1).
Shadow Collective AA5 BSE
Similar to the previous army this one also leverages the power of Maul but backs him up with AA5’s for healing and Black Sun Enforcers (BSE) as a second deadly threat. Brian Baer ran the above list at Gen Con and placed 2nd. The 3 main threats in this army have red saves and are backed up by two Backworld Medics, making them extremely hard to kill. This healing gives Maul and the BSE survivability after being dropped right on top of the enemy army. Just like the previous army, every unit in this army is in the top 15 units that have a positive effect on win rate (Table 1).
Blizzard Force Bikes
The trend continues; this Blizzard Force army contains only units in the top 15 units that have a positive effect on win rate (Table 1). The army leverages a huge bid combined with Vader and 4 Bikes to force favorable objectives like Bombing Run and Hostage Exchange. Stormtrooper Heavy Response Units also are highly effective for the low cost of 74 points which is reflected in the unit’s 70% win rate (Table 1). This army also comes with arguably the best bombardment in the game, Overwhelming Barrage, which can easily kill opposing Strike Teams. Finn P. played the above army at the Hannover WQ and placed in the top 4 with it.
Empire with High Velocity, Bounty Hunters, and/or Vader
These armies in particular are more varied between each list but I noticed a common trend. Empire armies that spam High Velocity (HV) and bring some mixture of bounty hunters were having lots of success. These armies are the first in this list that are not made up of only units in the top 15 (Table 1). The armies function more as a counter to the current meta. Pierce and High Velocity are good against Pykes and Black Sun Enforcers. All of these lists have at least 3 HV snipers. Some of these lists bring even more HV, like Izzy’s Iden Double Bounty army shown above. Izzy placed in 1st with this army at Gencon. Vader is also still highly competitive in the current meta. Pierce 3 and Force choke can cripple an entire Black Sun Enforcer unit. Dennis Jenkel won the Hannover WQ with the below Vader LAAT army, displaying Vader’s effectiveness.
The Way Forward
Each faction beside the Shadow Collective (all units over perform) and Republic (all units under perform) have some archetypes finding success in the new meta while others are struggling. Here I discuss the way forward for these factions based on the results of this analysis.
CIS
The Separatists had the biggest change in win rate after the release of Shadow Collective (Win rate Δ = -8%). Pykes and dodge spam heavily counter the previously dominant Kalani + 2 Spider Droids armies. This is clearly apparent in the win rates of these units. Both Kalani and Spider Droids are in the bottom 5 units when ranked in order of largest positive effect to largest negative effect on win rate (Table 2, STable 4). Separatists still have competitively viable options, but the armies do not look like what was previously dominant. Based on my analysis, Cad Bane, the T-Series, the AAT, and STAPs have the strongest positive effect on win rate in CIS in that order.
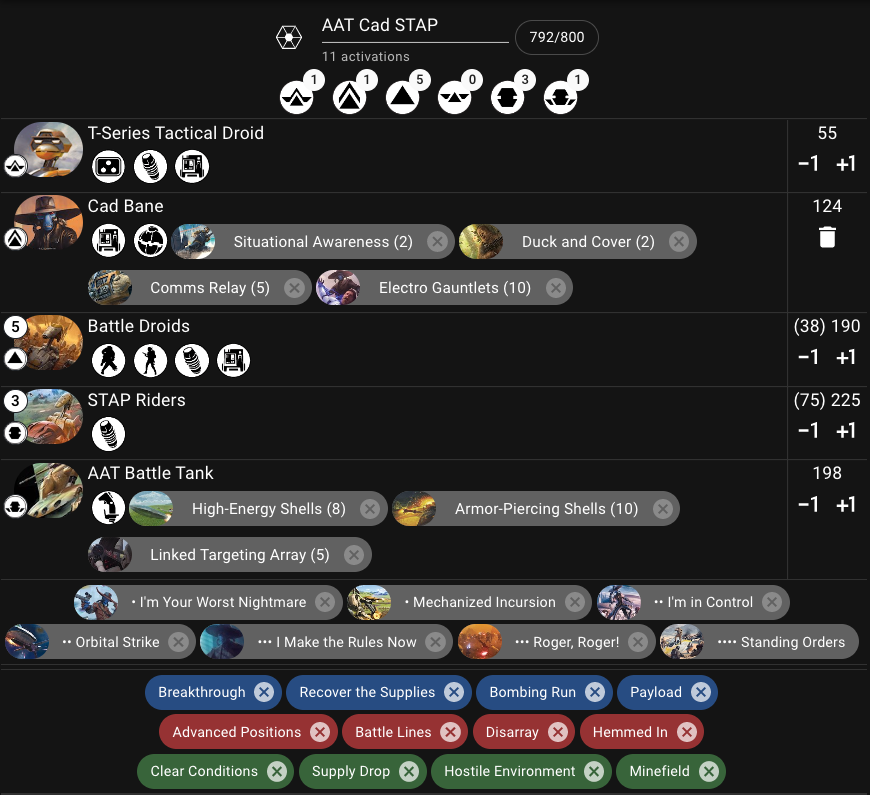
The above army can also take a deep bid like Blizzard Force, forcing opponents into tough objectives like Bombing Run and Recover the Supplies. The AAT brings High Velocity for Pykes, and perfect order control makes it so Cad Bane’s Independent: Dodge 2 triggers every turn while maintaining control over his activation timing.
REB
Rebels have a roughly 50% win rate currently and have a variety of army types that are finding success. The one common feature of top Rebel lists is Jedi Luke. Rebel dodge spam is still winning events but now there are two variations of the army. Each plays Luke but one opts for 4 DLT Rebel Situational Awareness (SA) Captains and 3 Snipers, with the other opting to take 3 DLT SA Captains, 2 Pykes, and 2 Snipers instead. The first brings more High Velocity to counter dodge spam, and the other brings 2 Pykes since they are currently over performing other units. While Rebel DLT Captains are slightly worse than Pykes, this army works because the extra HV snipers give an edge in the mirror. Also, the AA5 speeder truck is still a powerful unit. Some players are finding success with aggressive AA5 Rebel armies. The list below was played by Danny Trujillo who placed in the top 4 of Gencon.
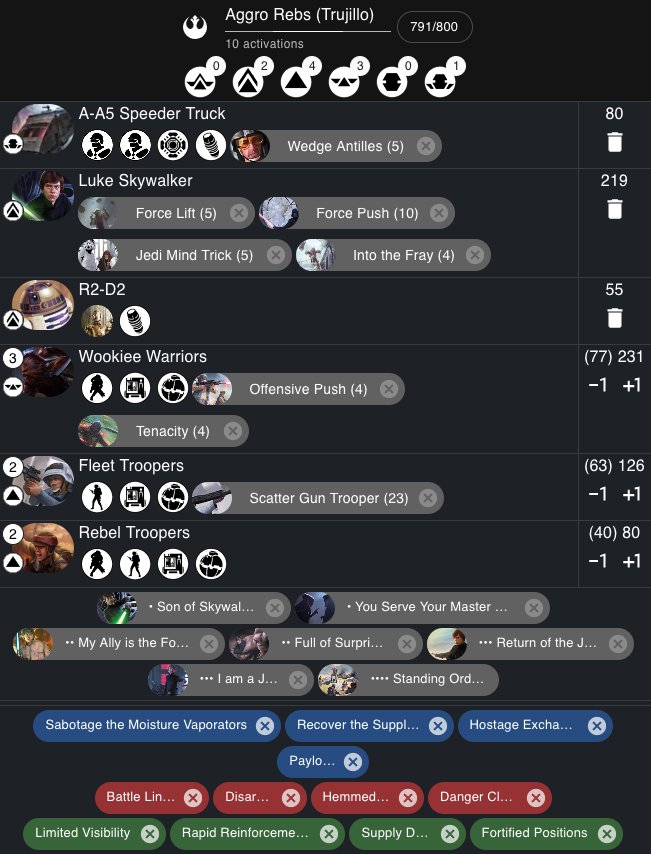
GAR
Republic is in a tough position currently. The best army they have is Anakin, Padme, and Pykes dodge spam, but this army is super vulnerable to both HV and Pierce which is very popular at the moment. You also have a disadvantage in the mirror match against either Rebel Dodge Spam or Shadow Collective Dodge Spam. Essentially the best Republic option is just a worse version of the other two. Not a single unit in the faction (beside Pykes) has a positive win rate. The top four units are Yoda Grand Master of the Republic with a 49.0% win rate, R2D2 with a 48.7%, Chewbacca with 46.3% and BARCs with a 45.1% (STable 4). This suggest a list like the following is your best bet as a Republic player. Taking a bid can help you get favorable objectives and deployments. Yoda is a virtual auto win on Hostage Exchange, and Secret Mission can win games when playing your blue player deployments.
Caveats
In this analysis there are underlying assumptions and potential confounding factors. Differences in player skill and co-correlation with faction/unit differences were not explicitly controlled for. This analysis treats each observation equally and assumes there is equal variance in player skill within each category (faction/units). The co-correlation of player effects and faction/unit effects can also be exacerbated by batch effects. For example, in each event 3+ rounds are played, meaning at a minimum a player plays the same list 3 times in a row causing an artificial correlation between player and the army/faction. These confounding factors can manifest in variety of ways such as: early adopter effects (top competitive players adopt the new units first, over-inflating the observed win rate) and contrarian effects (top competitive players seek to disprove popular sentiments, which can increase the win rate of underperforming factions).
Conclusions
Legion is currently in a statistically significant state of imbalance between the factions. The main two features that contribute the most to this imbalance are the over tuning of the Shadow Collective and weakness of the Republic faction. The first primary focus should be on increasing the cost of the Pyke Syndicate Capo (Unit and upgrade), Pyke Syndicate Foot Soldiers, Black Sun Vigo (Unit and upgrade), and Black Sun Enforcers. These generic units can be run en masse in the shadow collective (1-4 Commanders and 2-6 Core) exacerbating their effect on imbalance. This is supported by the fact that every faction can field the Pykes, and two factions can field Black Sun, but they are primarily an issue in the Shadow Collective battle force where they can be run en masse. The second primary focus should be on points reductions to the Republic faction. The Republic previously had the lowest win rate out of the four factions and the release of Battle Forces increased this discrepancy, making it a statistically significant difference. While the Republic was overtuned before the 2021 errata, the nerfs combined with the power creep of the Shadow Collective and Mercenary units have compounded, making the faction significantly weaker than all others. Points changes are especially important for the Republic considering there are no planned releases for the faction for the rest of the year. While each faction has differences in internal balance, Republic currently does not have viable competitive options. This contrasts with the Separatists who have a clear path forward. Their low win rate is a result of the previous top-competitive lists being countered by the Shadow Collective. Within the Separatist faction there are multiple units with a positive effect on win rates like the T-series and AAT, Separatist players need to start changing the archetypes they field. We found there was relative balance between the Empire, Rebels, and Separatists emphasizing the focus of future balance updates should be on the two clear outliers in the current state of the game.
Supplemental documents
Acknowledgements
This research was funded by the Fifth Trooper Network (TFTN). You can directly support our work through the TFTN Patreon https://www.patreon.com/thefifthtrooper and support Legion Stats development at https://ko-fi.com/drpowerslam. Statistical analysis, figure creation, and manuscript writing was done by Lucas J. Ustick. LegionStats data collection and pre-processing was carried out by Tue G. Bodersen. Invader League data collection and pre-processing was carried out by John R. Bushman. The manuscript was proofread and edited by Kyle D. Dornbos. I also want to thank all the members of TFTN for feedback on data products and the results.
17 Responses
Something I would be interested in is once all the invites to worlds are out, do an analysis of faction played by player over time. Games like this always have the opinion that top players just chase the meta but how often do they really play breadth (several factions) vs depth (one or two factions).
I had a somewhat related thought – I would imagine the data here are very weak, but some proxy for player quality would be interesting to add to the analysis.
When we look at statistically underperforming units, is it purely due to constraints in unit design, or could it also be due to selection clustering from high ranked players?
For example, if Pyke’s are anecdotally considered a good or popular unit, competitive players may gravitate to them. Players with less connection to the game, a weaker skill set, may not be aware of popular meta, so their unit selection could be more idiosyncratic.
In other words, less experienced players being more likely to pick less popular units might make those units appear weaker than they are.
I actually don’t think in my gut this would materially change the analysis, but still interesting!
I like the article. Here are my thoughts on it:
-For the article specifically, I would like to see ‘X’ games played as a baseline and that number should apply across the board equally. It’s a large disparity between 180 games for one unit and 33 for another. (This is a minor complaint)
-For SC, I would do several things:
-Pykes lose outmaneuver. Crits should get through and at least make them roll. This would be less of a feel bad on the first shot doing zero damage.
-Capo commander unit goes up to 50 points. The fact that this unit can give out one free dodge at the beginning of the turn and then multiple other tokens when he actually gets to go is too strong for 45 points.
-Redo the BF org chart to only give them 2 command slots. I’m not sure why they get 4 to begin with.
-For poor GAR, I would do the following:
-P1’s down 4 points.
-P2’s get Reliable 2 instead of 1.
-Arcs, change sniper to high velocity and give this unit 2 wounds each, lowering the model count to 3 . The more pierce and indirect damage released, the sadder one wound ‘elite’ models are, and it’s worth noting that every upcoming release has either pierce or Armor, things that traditionally GAR is weak against. (Check me on this, It’s tough to keep up with everything AMG has given us for future releases in the past few months, also a good thing!)
Imagine two heavys in a unit would be good.😏
Realy nice and interesting summary of the data.
Thanks Lukas!
Thank you very much for this massive analysis! Enjoyed reading it.
Whish you a good start in Germany and hope to meet you some day!
great read. thanks for all the work
While I’m sure the broad brush conclusions made will hold mostly true, there a couple of obvious things that would have been nice to consider given the attempt at real statistical analysis. Firstly referring to your own data, the biggest effect on win rate tables should be split out by faction/battleforce played in – while you will lose some level of significance at least the story of are pykes great (and where) vs is SC great is better defined. Second and most concerning is you have no control for player skill, having access to full identified data as well as a skill measure you could at least categorise you results by elo brackets. The story of better listbuilders (who tend to be better players) just rotated into SC and SC units and are enjoying a higher win rate is as viable as your conclusion without this information. On a tangent perhaps seeing how often factions score upsets rather than wins would be much more interesting. Finally, and this is much more subjective, the factions are so vast now that grouping them together (particularly with their battleforces) doesn’t make sense. Creating definitions for say the 3 most popular sub factions and preforming your analysis on each of those would yield more informative results, helping to exclude the play for fun lists as outliers (which we have a lot of) as it’s important for looking at this as a guide to competitive play. Without blowing out the length of the article or this comment I can see why you took the simplified approach you did. Thank you for bringing us some real stats beyond just the usual win rate percentages and averages we usually get from these types of articles.
This was so phenomenally well researched and presented. I love the idea of you doing these regularly! Great work Lucas! I hope AMG reads these and listens…
THIS IS AMAZING!! This is the balm for all hot takes. Lucas, you are doing high-quality work and I am eager to see more of this. Also, I hope people from AMG see this.
I’d like to know how specific player efficacy contributes to win rates for these units. As in, if only the top players are taking unit A, does that make unit A a top performer, or is it just that top players are taking unit A.
Were all the chi squared tests done for linear regression models? I’m surprised there isn’t a correlation with activation count, but I guess there’s probably not enough data to say anything about it at the moment
I did a Pearson’s Chi-squared test which compares count data based on grouping variables, in this analysis Faction, Activation Number, and Units in an army. So it was not based on a linear relationship, it compared expected counts to observed counts of wins and losses. I suspect activation did not have a significant effect because most games had 9-11 activations so the outliers did have lower or higher win rates but there were simply not many observations.
Any chance a link to the data could be added so others could look at the data?
Data is already linked at the bottom in the supplemental file with explicit methods as well.
Thank you for such an in-depth analysis of the current meta. It seems like a new rules and points update would do the game some good before end pf the year. I hope Atomic Mass Games reads your analysis and makes the adjustments to keep the game fun for everyone.
Another well written article by Lucas! If I may offer a few suggestions for future analysis reports and discussion, I think it would be helpful to disclose some of the major underlying assumptions that you’re making about the dataset and approach and also make at least a statement about the other possible contributing factors that while they clearly can’t be supported by the data, also can’t be ruled out because of the constraints imposed on the analysis.
Some examples of what I’m talking about here are things like meta bias (which is partly addressed by which units did not have enough play time to make the cut) and meta learning curves. There appears to be an assumption of perfect distribution of information over the entire meta, but is two months really enough table time (real or virtual) for the meta as a whole to adjust to the new lists AND play styles? If yes, I’d like to know the assumption for why. If no, at least address the possible variance point even though it won’t change your conclusions. As you or others do more of these, it’d be good to settle on some common clear parameters in the results over time that can be used for covariance by anyone who wants to do these types of analysis.
Lastly, and this has been mentioned by others but I’ll reinforce: skill level/rule of cool combined with a very high influx of new players. I don’t pretend to have an answer for this, but I’d like to understand what assumptions can be made in your approach and analysis for cases like “I love republic, I play them all the time, but I lose because I’m new” vs “faction is in a bad space”. It may be as simple as “new players aren’t submitting their lists to legion stats”- how do we know? or is it an assumption? Things like that.
You’ve already put so much time and effort into putting together a thorough analysis and I applaud you for that. I also understand that every discussion point added to a report that doesn’t immediately and clearly support it’s conclusion is eating up the attention of the reader, however I do think a simple assumptions section added to the introduction or before the results to touch on these broader points would be helpful in addressing some of the common talking points I’m certain will pop up as rebuttals to future reports like this.
Other than that, I can’t wait to be at the opposite end of the table from you again Lucas!
Thanks for all the thoughtful feedback Nick! I miss playing with the OC crew hopefully will be able to make it out to the SoCal open next year to see everyone.
Great article Lucas, was really interesting to read something so in-depth as a data enthusiast myself.
I had a couple of questions about the hypothesis testing here (the Pearson’s chi squared section).
1. My understanding was one of the assumptions required observations to be independent of each other. However in this case knowing that player 1 (and hence faction 1) won a game implies the opposing player/factions lost, so I’m not sure if this assumption holds. What are your thoughts on this?
2. Is there any way to control for the distribution opposing factions while dong this analysis? For example, what I mean is if GAR played majority of their games against Shadow Collective then the faction win rate calculated here may look a lot worse than if there was a uniform distribution of opponents.